Sam Fok, co-founder and CEO of silicon startup Femtosense, explains how Arm Flexible Access for Startups enabled the development of a neuromorphic AI chip
One classical pathway to technology success runs from university research to commercial startup. It’s an exciting journey in which a small group of grad students takes a spark of innovation from research and kindles it into a technology that they hope will transform the world.
That journey has its challenges as the technology moves from the lab into the rigorous and sometimes unforgiving world of product development. And it’s a journey my co-founder, Alexander Neckar, and I embarked on two years ago out of Stanford.
Our startup, Femtosense, emerged from work we did in Stanford’s Electrical Engineering graduate program on a project called Braindrop—a mixed-signal neuromorphic system designed to be programmed at a high level of abstraction.
Neuromorphic systems are silicon versions of the neural systems found in neurobiology. It’s a growing field, offering a range of exciting possibilities such as sensory systems that rival human senses in real-time.
Over the past two years, we’ve nurtured our startup to develop the aspects of the technology with commercial potential. We’ve taken the original concept and built a neural network application-specific integrated circuit (ASIC) for the general application area of ultra-efficient AI. As a start, we want to enable ultra-power-efficient inference in embedded endpoint devices everywhere.
Why did we venture down this path? Data centre technology is well-developed and has different technical challenges than edge technology. Further, consumers and market competition are pushing for edge and endpoint devices with ever-increasing capability. AI can and should be deployed at the endpoints when feasible to reduce latency, lower computing costs, and enhance security.
Area and power major considerations in neural networks
But designing such an ASIC (any ASIC really) is a unique challenge in today’s ultra-deep submicron world. For one thing, area and power considerations loom large. Neural networks are a different animal than classical signal processing. Designing an ASIC or system-on-chip (SoC) for neural networks presents a different challenge than, say, designing an efficient DSP or microcontroller.
Neural networks have a lot of parameters, are quite memory intensive, and have a lot of potential for energetically expensive data motion. Of primary concern is the question of on-chip versus off-chip memory. Off-chip memory provides excellent density, allowing for bigger models, but accessing off-chip memory costs a lot of energy, which often puts such systems beyond the power budgets of the envisioned initial applications.
So, when you’re looking at ultra-power-efficient endpoint embedded solutions, you will want to put everything a single die, but then you will hit area and cost issues. This is where algorithm-hardware codesign comes into play; the two really must be done together.
You can’t just naively take an algorithm and map it down to a chip. It would cost too much energy or money or not just perform well. You have to think carefully about how to make that algorithm efficient. That’s the hardware and algorithm co-design challenge. We spend a lot of effort on the algorithm side to fit everything on-chip, and, once it’s on-chip, to map the algorithm onto the chip’s compute fabric.
As we design, we’re exploring many potential applications because we want to apply our technology as widely as possible. The market is not nearly as black-and-white as endpoint-and-cloud terminology suggests.
We see it as a continuum. It’s not like you’re either in datacenters or in tiny battery-powered devices. There are many nodes across the spectrum—everything from on-prem servers, to laptops, phones, smartwatches, earbuds, and even sensors out in the middle of nowhere with no power source could use more efficient neural network compute.
We want to deliver ultra-efficient compute. To us, this is our primary mission. When you’re in tough environments with tight requirements, that’s when you’re going to want to use our technology.
Arm Flexible Access for Startups gave us the design flexibility
We’re a small team for now, so we need to focus on our strengths. There’s the core hardware accelerator, the software that goes with it, and the algorithms. Then, there’s technology to take that value and serve customers. This is where we’re very excited to be working with Arm and the Arm Flexible Access for Startups program.
To integrate with customer designs, you need an interface to handle communications and off-load a bit of compute. This is not something we think about day-to-day in terms of our core engineering or IP. This is why it’s paramount to have a well-established, reliable partner like Arm to work with because you don’t have to reinvent everything or spend effort educating the market. Everyone knows Arm, and Arm is a well-known path to integration. Arm solves one of our biggest commercial challenges, and that’s why working with Arm is key.
One of the attractive elements of the Arm Flexible Access for Startups program is the ecosystem support. The ecosystem reduces barriers to adoption, which in turn drives innovation. When you’re planning projects, you need clear and accessible specifications and information about which products do what.
Making actionable information available upfront without huge outlays and with the ability to evaluate different IP and run experiments in the sandbox is huge for us. The program’s pricing models are much more aligned with how startups grow than traditional IP vending.
Standing at the beginning of the SoC integration path, we have an exciting journey ahead, and we’ll have more interesting perspectives the deeper we get into it. We have the initial design and are moving toward an ASIC implementation, making the Arm Flexible Access for Startups program an important tool to have.
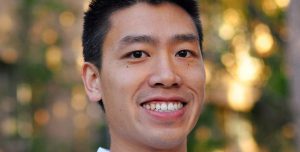