Predictive maintenance is the latest paradigm in maintenance and with emergence of IoT and big data it is redefining the way manufacturing industry operates. It is an important component of Industry 4.0and Smart Factory vision and mainly consists of increasing efficiency by planning maintenance activity based on detection or prediction of failures in the process or equipment based on data analytics methods. Amongst the many benefits of PdM compared to reactive and preventative maintenance is the ability to plan resources and alternate paths for processing so that the production doesn’t stop and also extending the uptime of the equipment. In the context of Semiconductor Manufacturing, the work item is a thin disc of Silicon referred to as wafer. The wafer undergoes a series of highly controlled and complex processes such as cleaning, deposition, patterning, etching, metallization, measurement and electrical testing. The wafer contains many “chips” patterned on it and the percentage of good chips at the end of all processes indicates the wafer yield. Nearly every electronic device that was ever created started off as a chip on the wafer. These processes are highly interdependent and the equipment used are often embodiments of state-of-the-art technology. Predictive maintenance also helps reduce wastage and avoid abrupt breakdowns of the process flow. It is especially critical in Semiconductor Manufacturing as the processes are highly dependent and cost intensive. In addition, it also significantly impacts the productivity and yield.
Some of the requirements for successful PdM set up are:
- Data quality: Sensor data carries important signatures of the health of the equipment, process as well as the wafer. It is imperative that the data be accurate and free of noise or influence by extraneous factors as it forms the main input for rest of the PdM set up.
Pervasive sensing: Sensors convert the physical characteristics of equipment and the process to digital domain. In order to get the comprehensive picture of the physical factors, it is important to cover all the important interactions between wafer, equipment and process. This requires increasing the sensor coverage to monitor pressure, temperature, vibrations, humidity, flow of gas, power etc. at multiple locations.
In case of using image as data for anomaly detection or root cause analysis in semiconductor manufacturing, as we move into smaller geometry process nodes, imaging based fault detection techniques require higher resolution cameras to be able to identify the smaller features and any anomalies. Also, a higher frame rate is required to be able to keep up the normal movement of the objects under test. In addition, camera or sensor should be able to adapt to the environment of the manufacturing and be able to capture high quality images in any lighting conditions. It is challenging to upload and store this amount of data in real-time. Therefore, an edge AI platform is highly desirable which can analyze and filter the required for further analysis. - AI modeling: The factors that affect the wafer or the proper functioning of the equipment are quite complex. However, the data available is huge. This is good case for utilization of AI modeling that can learn the complex and non-linear relations between the physical state of the process and sensor data. The AI models play an important role of detecting and predicting anomalies, bad process conditions and remaining useful life of the equipment or its module.
The accuracy of the models becomes an important pillar of PdM. In addition to creating the models, a strategy for time based model updation is required since the relation between different process parameters change over time and is highly dependent on the placement of sensors and equipment layout. In addition, physics and domain knowledge infusion in AI models is also desirable to improve accuracy and gain from the experience of experts.
Most of the recent research effort in PdM has been in the area of creating advanced and complex AI models. Another field of interest in recent years has been explainable AI whereby the predicted failure modes and the reasons for the same can be described in terms of physical factors that can be easily understood by the stakeholders.
Contributory Authors
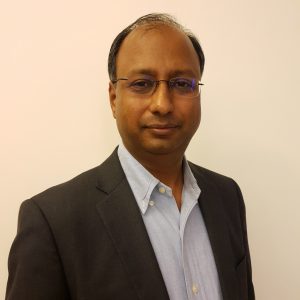
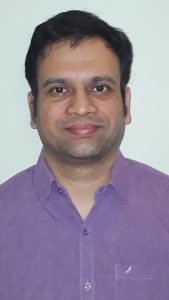